Authors’ note: Product teams at Facebook rely on research along with other external factors to design and build products. This article discusses research conducted by Facebook's Privacy Research Team to better understand people's privacy concerns.
Abstract
We conducted a survey to measure privacy concerns across a range of popular apps and topics. The percent of respondents concerned about each privacy topic that we measured was similar across all of the apps.
The results suggest that people’s privacy concerns for individual apps might have more to do with their beliefs about privacy topics in general than they do with their beliefs about the apps themselves or their experiences using those apps. For example, whether someone is concerned about Facebook using information to personalize ads may largely reflect whether that person is concerned about ad personalization by any app.
We discuss the implications for how companies might try to address users’ privacy concerns for their products in light of these results.
Report
Intuitively, people’s privacy concerns for an app should be related to features of the app itself, including what data the app collects about users, how the app uses that data in various features, and the app’s privacy policies. At Facebook, we wanted to learn how people’s privacy concerns for our apps compared to their privacy concerns for other apps that differed on those factors. We conducted a survey to measure privacy concerns across a range of apps and products, and what we learned surprised us: The percent of respondents concerned about each privacy topic that we measured was similar across all apps that we investigated.
The results suggest that people’s privacy concerns for apps are not strongly related to the apps themselves; instead, privacy concerns for individual apps actually reflect people’s overall beliefs about apps and technology in general. In this article, we’ll describe the research we conducted and discuss the implications for how companies might try to address users’ privacy concerns for their products.
Method
Facebook’s Privacy Research Team conducted an unbranded survey in five countries (United States, Brazil, Germany, India, and Indonesia). A total of 25,467 respondents reported their familiarity with 12 popular apps and companies (henceforth “apps”). Each respondent then reported their privacy concerns for two randomly-selected apps, among apps they were familiar with. Specifically, we asked about the 10 privacy topics that represent the top-of-mind privacy concerns for data collection, data use, and data access among people in the surveyed countries (Hepler & Blasiola, 2021). We measured privacy concerns using the Privacy Beliefs & Judgments (PB&J) scale (Hepler, 2021). PB&J asks respondents two questions for each privacy topic: (1) Whether respondents believe a privacy outcome is happening, and (2) whether respondents judge that outcome to be good or bad. Responses are labeled as being concerned about the topic if they say they believe it’s happening and that it would be bad for it to happen. For example, here is how we measured concern related to apps using information to personalize ads:
1. Do you believe [app] uses information about you to determine what ads to show you on [app]? [Yes, No]
2. If [app] did that, would it be good or bad? [Good, Neither good nor bad, Bad]
Respondents who said “Yes” to question 1 and “Bad” to question 2 were labeled as “concerned” about this topic for this app. Respondents who said “No” to question 1, “Good” to question 2, or “Neither good nor bad” to question 2 were labeled as “not concerned”. In other words, the PB&J approach defines respondents as concerned if they believe an app engages in a privacy practice that they believe is bad to do. For additional information on our research methods, see Appendix A.
Results
The results for all five countries in this research are similar and lead to the same conclusions. To simplify our discussion and data presentation, we’ll focus on the results for US respondents here. The results for the other countries are in Appendix B.
This figure shows the percent of respondents who were concerned about each privacy topic for each app in the US sample. The apps are tightly clustered together across the 10 privacy topics. For most topics, the apps are within ±5 percentage points of the topic’s average score. As we move from topic to topic across the figure, concern for each app moves up together or moves down together, and they move by roughly the same amount. Across topics, apps cluster around a minimum of 25% concerned for Topics 1-3, and a maximum of 65% concerned for Topic 10. So the between-topic range (25% to 65%) is much larger than the between-product range for each topic (±5%). In other words, privacy concerns are more related to the topic than they are to the particular product.
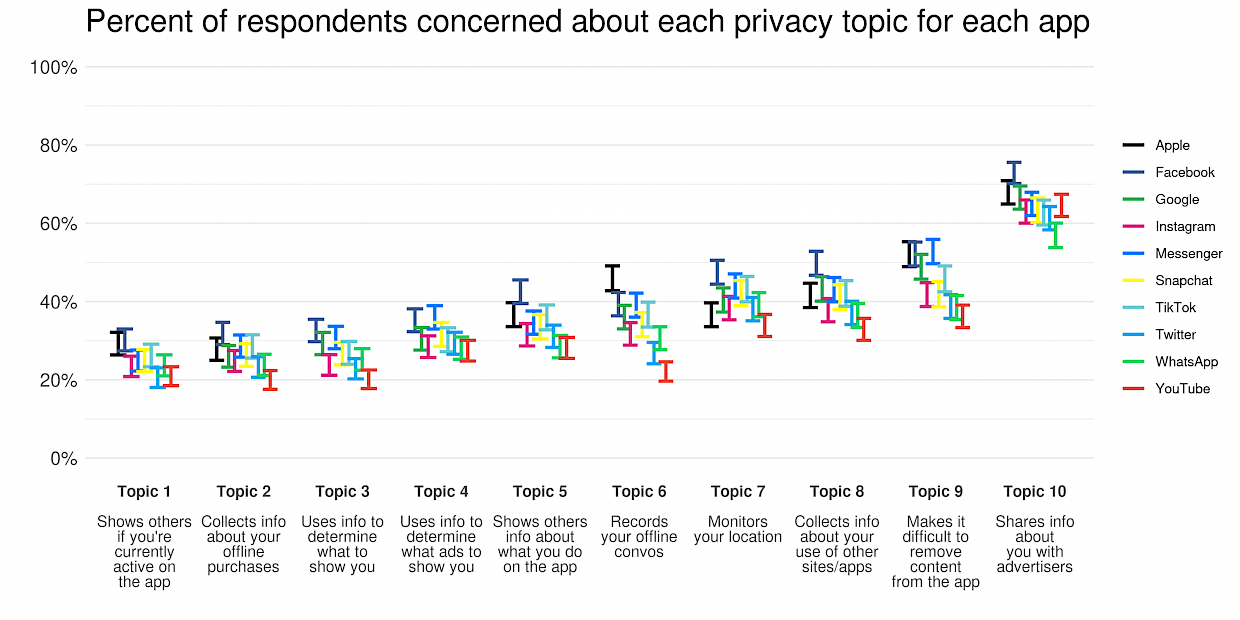
Figure caption: Error bars represent 95% confidence intervals (CIs). If two error bars don’t overlap, the values are stat sig different from each other.
There are also reliable differences between apps. For example, across topics Facebook is consistently highest, Snapchat is consistently in the middle, and YouTube is consistently lowest. This consistency suggests there may be an overall app effect for privacy concerns, in which concerns are shifted up or down in general for an app regardless of what privacy topic is considered. We can explore this app effect in two ways. First, we can center the data within-topic to see if the app effect has a reliable magnitude across topics. We’ll do this by subtracting the average privacy concern score for Topic 1 from each of the individual app’s scores for Topic 1, and then repeat this for the remaining topics.
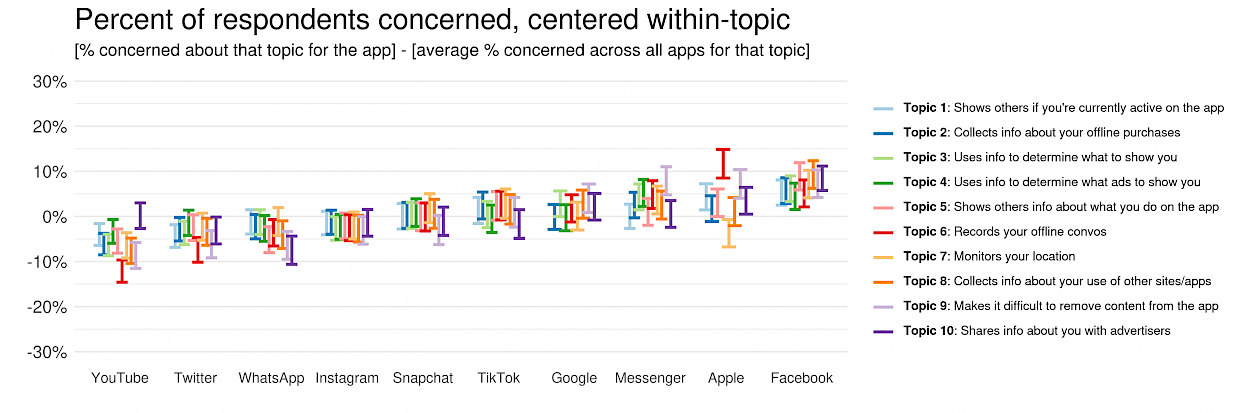
Figure caption: Error bars represent 95% confidence intervals (CIs). If two error bars don’t overlap, the values are stat sig different from each other.
Looking at the within-topic centered data, we can see the overall app effect is constant across all topics (except for four outliers — Apple for Topics 6 and 7, YouTube for Topics 6 and 10; it’s worth noting that when comparing 100 data points, we’d expect to see a few differences just by chance even if no true differences exist between apps). For example, Facebook scores 7 percentage points higher on concern for all 10 topics relative to the average, Instagram scores 2 percentage points lower for all 10 topics, etc. This means that the effects of app and topic are mostly independent of each other, and there appear to be flat, fixed differences between apps across all privacy topics we’ve measured. For Messenger, that fixed difference is 3%, which means that if we want to know how much higher concern is about privacy for Messenger relative to the other apps, we don’t even need to know what privacy topic we’re talking about — it’s always going to be about 3% higher. For Instagram, the fixed difference is -2% — concern is always going to be about 2% lower. The table below shows the average fixed difference for privacy concerns for each app in the US.
Table 1. Average difference in concern scores for each app.
YouTube | Snapchat | TikTok | Messenger | Apple | |||||
-6% | -4% | -4% | -2% | +0% | +1% | +2% | +3% | +4% | +7% |
Table caption: Higher positive values indicate more people are concerned relative to average.
Importantly, different people are familiar with the different apps we investigated, and that could be responsible for some of the observed differences between apps. For example, if people aware of Facebook are simply more likely to be concerned about any privacy topic in general relative to people aware of YouTube, this could contribute to the effects we see. So the fixed differences could be due to (a) differences in how consumers perceive the apps, (b) differences in the population of who’s familiar with each app, or (c) both. Regardless, we can confidently say that different apps are consistently rated differently across all 10 privacy topics by the people who are familiar with those apps.
The second way we can explore this overall app effect is to center the data within-app. We’ll do this by subtracting the average privacy concern score for an app across all 10 topics from each of the app’s scores for Topics 1-10. This will allow us to see whether the relative prevalence of concern for each privacy topic is the same once we account for app-specific effects.
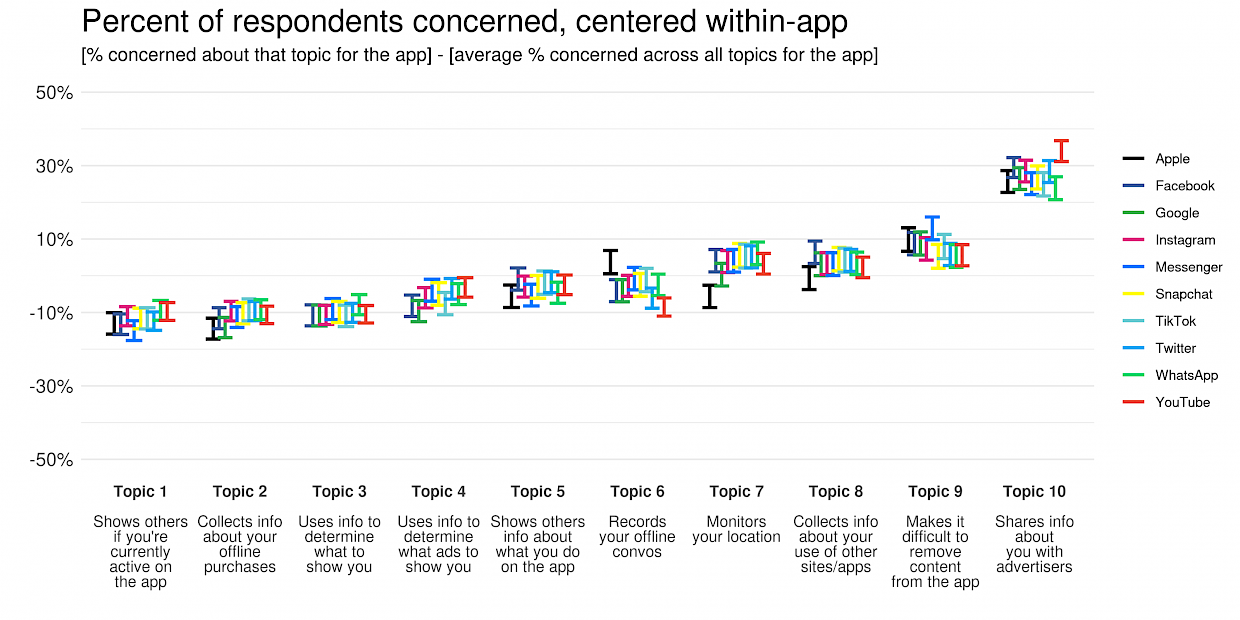
Figure caption: Error bars represent 95% confidence intervals (CIs). If two error bars don’t overlap, the values are stat sig different from each other.
After adjusting for the baseline level of privacy concern for each app, the between-app differences nearly disappear and the relative prevalence of privacy concerns are virtually identical across all 10 apps. The only notable exceptions out of all 100 data points in the figure are the four outliers mentioned earlier (Apple for Topics 6 and 7, YouTube for Topics 6 and 10). In other words, once we adjust for factors like brand effects and population differences, rates of privacy concerns are the same for all the apps.
Implications
The apps we explored in this research differ substantially in terms of the data they collect about users, how they use that data, and what’s included in their privacy policies. And yet, the prevalence and rank order of the 10 privacy concerns that we measured was nearly identical across all the apps. And when the prevalence did differ between apps, it was by a small, fixed amount for all 10 topics that reflected differences in user base or overall brand reputation. Taken together, these results suggest that people’s privacy concerns for individual apps might have more to do with their beliefs about privacy topics in general than they do with their beliefs about the apps themselves or their experiences using those apps. For example, whether someone is concerned about Facebook using information to personalize ads may simply reflect whether that person is concerned about ad personalization in general, regardless of which app is doing it.
But why would privacy concerns have more to do with the topic rather than the individual apps? The reasons for this may stem from how people form their beliefs and understanding about privacy overall. For example, two such reasons are:
People sometimes rely on a general understanding of technology when trying to understand specific apps. To illustrate this, let’s consider the topic of ad personalization. For some people, ad personalization is a bit of a black box — although they understand that information about them goes into the box and that a personalized ad comes out of the box, they don’t know exactly what happens in between. And for some people, the box is too technical, complex, or boring to want to spend time learning about. So instead of learning what actually happens, they rely on their intuition and come up with their best guess. For some people, this leads them to rely on myths about how technology works - e.g., incorrectly assuming that some personalized ads could only be possible if a company was selling their data or spying on their offline conversations. And those myths are app-agnostic, so they may be used when attempting to understand any app.
Generalizing privacy concerns across different apps can be a functional strategy. To illustrate this, let’s consider individuals who suspect that some apps they use sell data about them to advertisers. Because some of these individuals won’t want to invest the time and effort to learn about the specific details of every app’s data sharing policies, it can actually be easier to just assume any given app might be selling their data to advertisers if they suspect any apps do it at all. By assuming this, these individuals can act in a way that keeps them safe no matter what app they’re using. If they took the time and effort to keep track of the details of every app’s policies, they wouldn’t need to make this assumption — they could behave differently on each app based on what the app’s privacy policies were. But some people won’t track this information, so it’s just easier for them to assume all apps do something they believe is bad if some apps might do it. In other words, generalizing concerns across all apps can be a functional strategy to avoid privacy outcomes someone perceives to be bad.
So what should companies do if people’s privacy concerns about their apps aren’t entirely about their apps per se? We’ve identified three potential strategies for consideration.
Strategy 1: Clarify how your app’s practices differ from users’ general privacy beliefs. For example, let’s consider concerns about monitoring users’ location. Some apps don’t do this, and many other apps provide users with controls to toggle location data on or off. In these cases, apps might be able to address users’ concerns through in-product education that clarifies the app doesn’t collect location data or that proactively provides reminders and tutorials for how users can toggle location data off in case they’d prefer to disable it.
Strategy 2: Educate users about technology in general. In some cases, it may not be sufficient to simply tell users your app doesn’t engage in a certain privacy practice. For example, let's revisit the personalized ad discussion from above. If some people believe the personalized ads they see could only be possible if a company sold their data to advertisers, then simply telling those users that your app doesn’t do that might not be believable because those users genuinely might not know how else it would be possible for their ads to have been personalized. So in addition to clarifying that your app doesn’t sell user data to advertisers, you may need to explain how ad personalization works in general in order to help users understand that the level of personalization they experienced in those ads was in fact possible through other means.
Strategy 3: Push for standardization in certain privacy practices across the tech industry, and clearly communicate those standards to consumers. If industry-wide privacy standards are set and enforced well, they could help consumers feel confident about the privacy experiences they can expect by default when using apps. In turn, this should help make consumers less likely to rely on myths or generalizations that result in false beliefs about apps engaging in undesirable practices when they’re not actually doing so. People already seem to hold similar beliefs across apps today, so standardization would also help the tech industry as a whole meet consumers where they are in terms of their understanding of privacy practices.
Although we laid out three potential strategies for addressing privacy concerns, it remains to be seen which are more or less effective at addressing privacy concerns across different privacy topics and different user groups. It’s also possible that additional strategies will need to be developed beyond these three in order to fully address users’ concerns. Ultimately, the best way to address users’ privacy concerns is still an open and challenging question across the tech industry that’s going to require exploration and testing. This research provides insights that we believe can help power those important explorations for companies like Facebook that are committed to honoring people’s privacy.